Research
How much carbon will escape from the (sub)Arctic in future climate?
How do the multitude of ecosystem processes, driven by plant growth, microbial activities and soil characteristics, interact to determine (sub)Arctic soil carbon storage capacity?
These are the central research questions of FutureArctic.
They are addressed at a unique location. The ForHot (www.forhot.is) site in Iceland offers a geothermally controlled soil temperature warming gradient, where Subarctic ecosystem processes are affected by temperature increases as expected through climate change.
Given the strong urgency of tackling the climate challenge and the particularly important role herein of (sub)Arctic ecosystems, a rapid assessment of the ecosystem and ambient processes at the ForHot site will provide potentially crucial insight in future carbon cycling.
If this knowledge is to be applied in the climate change challenge, significant advances should be made on short time scales, including an unprecedented search for unknown interactions within the ecosystem. FutureArctic will achieve this challenge by adopting the fast advances made in the field of machine learning and artificial intelligence (AI), unmanned aerial vehicles (UAV) and (remote) sensor technology into environmental research at the ecosystem scale, into a new concept of an ‘ecosystem-of-things’.
FutureArctic embeds this research challenge in an inter-sectoral training initiative for early stage researchers, aiming to form ‘ecosystem-of-things’ scientists and engineers, in order to:
- Pave the way for generalized permanently connected and high-throughput data acquisition systems for key environmental variables and processes. Technological advances in scientific instrumentation in regard to both sensor capabilities and connectedness are necessary to achieve permanent analysis and data integration. The interaction with industry partners to achieve these advances is a key to success (WP2).
- Initiate a new machine-learning approach to analyse large high-throughput environmental data-streams. ForHot, with its unique and large subarctic warming gradients, becomes a pioneer project for the ‘ecosystem-of-things’. Machine learning analysis procedures will be implemented (WP3) to complement hypothesis-based environmental research (WP1). Will big data and machine learning algorithms inspire new ways of thinking into environmental science and ecology, complementing traditional hypothesis-based-research that does not make full use of valuable information contained in data?
- Create an ethical / philosophical framework for the implementation of machine-learning/artificial intelligence approaches into environmental science. What are the potential implications for traditional science, what are the caveats and pitfalls?
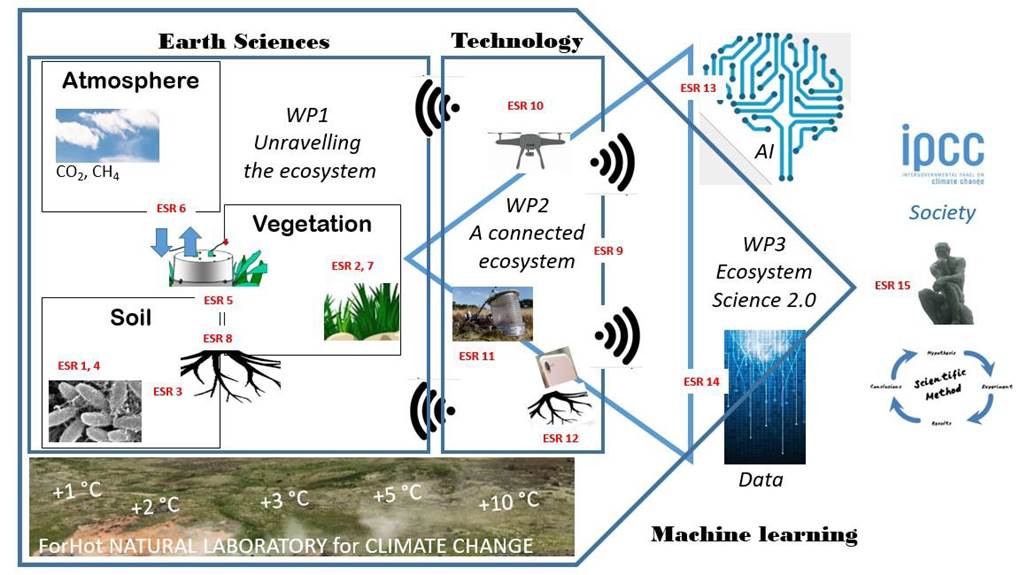

This project has received funding from the European Union's Horizon 2020 framework programme for research and innovation under grant agreement No 813114.